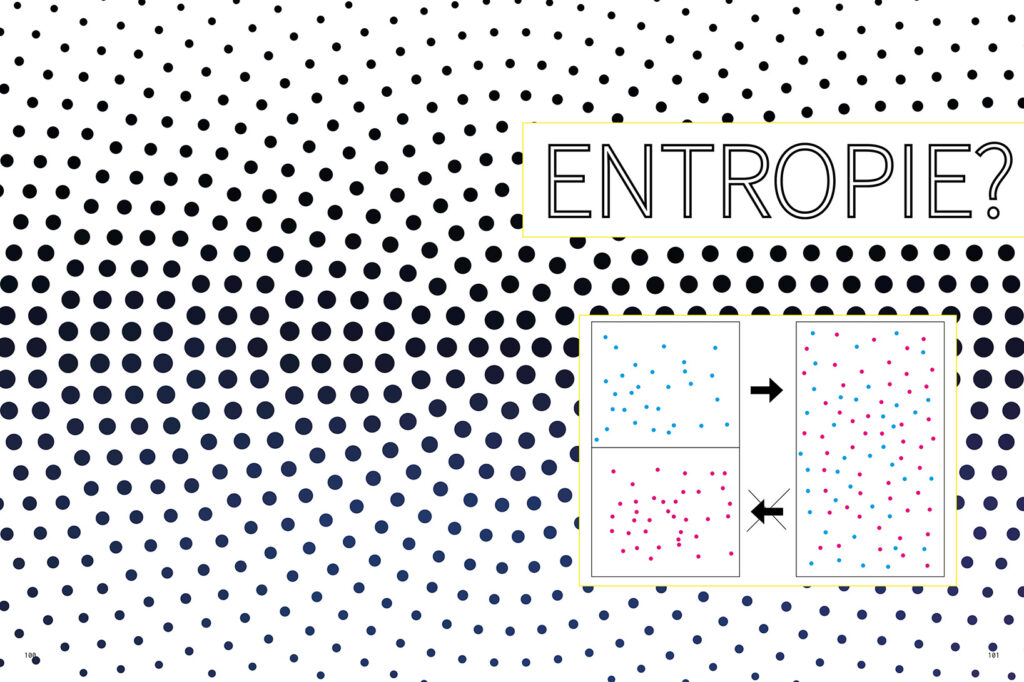
The term ‘entropy’ (Entropie) is a fundamental concept in physics, but also has far-reaching implications in other scientific fields such as information theory, biology and even social sciences. In this model, it is one of the three key phenomena that determine the dynamics of reciprocal resonance spaces. In addition, there are the phenomena of synchronisation and emergence, which possibly promote the achievement of a new stage of development, the creation of a team spirit or other entities in an interaction that is still difficult to define. (Main hypothesis)
Definition:
Entropy is a measure of the degree of disorder or randomness in a system. In thermodynamics, it describes the number of possible microstates of a system, while in information theory it is a measure of uncertainty or information content.
Discussion:
- Thermodynamic Perspective:
The term ‘entropy’ was introduced to thermodynamics by Rudolf Clausius in 1865 [1]. The second law of thermodynamics states that entropy can never decrease in a closed system. Boltzmann (1877) provided a statistical interpretation of entropy, linking it to the probability of microstates [2].
- Information Theory Perspective:
Shannon (1948) transferred the concept of entropy to information theory, where it is a measure of the information content or uncertainty in a message [3]. This connection between physical and information-theoretical entropy has profound implications for our understanding of information and physics.
- Entropy and Order:
Schrödinger (1944) discussed the role of entropy in biological systems in his influential work ‘What is life?’. He argued that living organisms absorb ‘negative entropy’ from their environment in order to maintain orderly structures [4].
- Entropy in Complex Systems:
In the theory of complex systems, entropy is often discussed in connection with self-organisation and emergence. Prigogine and Stengers (1984) showed how systems far from thermodynamic equilibrium can generate ordered structures through entropy production [5].
- Entropy and Evolution:
Brooks and Wiley (1988) argue that biological evolution can be understood as an entropy-driven process in which the increase in entropy is accompanied by an increase in biological complexity [6].
- Entropy in Cognitive Science:
In cognitive science, the concept of entropy is increasingly used to explain cognitive processes. Friston (2010) proposed the ‘free energy principle’, according to which biological systems strive to minimise their free energy (closely related to entropy) [7].
- Social Entropy:
Bailey (1990) applied the concept of entropy to social systems and argued that social entropy could be a measure of inequality and disorder in societies [8].
- Entropy and Arrow of Time:
The increase in entropy is often associated with the direction of time. Eddington (1928) coined the term ‘arrow of time’ and linked it to the increase in entropy [9].
- Entropy and Information:
Landauer (1961) demonstrated a fundamental link between information and physics by arguing that the deletion of information is always associated with an increase in entropy [10].
- Entropy in AI Research:
In AI research, entropy is used as a measure of uncertainty in models. Entropy-based methods play an important role in areas such as machine learning and decision-making under uncertainty.
- Entropy and Creativity:
Some researchers have suggested that creative processes can be understood as a balance between order (low entropy) and chaos (high entropy). Kaufman and Kaufman (2004) discuss how optimal levels of entropy can lead to creative breakthroughs [11].
Summary:
Entropy is a versatile and fundamental concept that goes far beyond its original thermodynamic meaning. It provides a bridge between physical, information-theoretical and biological concepts and helps us to understand processes of order and disorder in different systems.
In relation to our earlier discussions of resonance spaces and the emergence of collective representations, entropy can be understood as an important concept to describe the dynamics of information flows and the emergence of order in social and cognitive systems. It also provides a conceptual framework for understanding how systems (be they biological, social or artificial) process information and adapt to their environment.
Combining entropy with concepts such as emergence and synchronisation opens up new perspectives on the emergence of complexity and order in different systems, from neural networks to social structures and possibly even artificial superintelligences.
Literature:
[1] Clausius, R. (1865). Über verschiedene für die Anwendung bequeme Formen der Hauptgleichungen der mechanischen Wärmetheorie. Annalen der Physik, 201(7), 353-400.
[2] Boltzmann, L. (1877). Über die Beziehung zwischen dem zweiten Hauptsatz der mechanischen Wärmetheorie und der Wahrscheinlichkeitsrechnung respektive den Sätzen über das Wärmegleichgewicht. Wiener Berichte, 76, 373-435.
[3] Shannon, C. E. (1948). A mathematical theory of communication. Bell System Technical Journal, 27(3), 379-423.
[4] Schrödinger, E. (1944). What is life? The physical aspect of the living cell. Cambridge University Press.
[5] Prigogine, I., & Stengers, I. (1984). Order out of chaos: Man’s new dialogue with nature. Bantam Books.
[6] Brooks, D. R., & Wiley, E. O. (1988). Evolution as entropy. University of Chicago Press.
[7] Friston, K. (2010). The free-energy principle: a unified brain theory? Nature Reviews Neuroscience, 11(2), 127-138.
[8] Bailey, K. D. (1990). Social entropy theory. State University of New York Press.
[9] Eddington, A. S. (1928). The nature of the physical world. Cambridge University Press.
[10] Landauer, R. (1961). Irreversibility and heat generation in the computing process. IBM Journal of Research and Development, 5(3), 183-191.
[11] Kaufman, J. C., & Kaufman, A. B. (2004). Applying a creativity framework to animal cognition. New Ideas in Psychology, 22(2), 143-155.